Watch the Video
Data analytics for urban mobility planning is necessary to develop efficient resource management and infrastructure in cities and peri-urban areas. Riga (Latvia) deals with an increased number of commuters from the outskirts and neighbouring municipalities to the city as people have relocated to areas with lower population density. This outflow has a direct impact on the transportation system and creates the need for AI-driven decision-making tools for mobility planning. To optimise the transport network and its efficiency, make public transport more attractive to the user, adapt to changes and forecast future demand, real-time data analytics of travel behaviour is essential.
How EMERALDS will address the challenge
Aggregating a complete and versatile dataset on people travel habits is challenging, requiring the validation of the entry, exit and transfer points of the trip (to determine the travel route), and the participation of multiple stakeholders, in the dataset development. To increase the accuracy and the value of the dataset, all mobility modes are required. A preliminary algorithm for joining the datasets and geolocation validations provided by Riga Traffic has been developed and tested by Grupa93. However, it is essential to improve the algorithm and real-time data aggregation and analytics methods to evaluate longer time periods (and not only one day travel behaviours).
How it will work
This use case will develop a traffic data analysis toolset to analyse and forecast passenger travel behaviour and optimise the public transport network, making it more attractive to the user Currently, it is possible to geo-locate close to 70-80% of all ticket validations/entry points and 30-40% of all exit points. EMERALDS aims to improve the algorithm to geo-locate 100% of the validations and improve its accuracy. The trip destination is determined by geo-locating the next entry point and searching for the possible exit points for previous leg in a near radius. Thus, performing joins with real-time or calculated data on private car traffic, total cost of mobility per network segment could be assessed and used for planning. Combined with vehicle movement data, the algorithm will be able to calculate time losses via schedule and via free-flow conditions. For trips with no subsequent trips, AI could be used to infer additional trip characteristics (most popular destinations, probability distribution, etc.). The toolset will be attractive to municipalities and public transport providers to assess the passenger travel habits and to evaluate the multimodality potential.
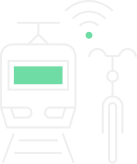