Watch the Video
Traffic congestion is a global and growing problem in cities that many of us experience every day. As the numbers of commuters grow, so do the traffic jams and wasted time and energy as travellers try to reach their destinations. Multi-modal traffic management is an important tool to promote a modal shift to more sustainable mobility options, prioritizing active modes and public transport and reducing mobility spatial footprint. The Intelligent Transport Systems industry has been working on the development and deployment of network-wide multi-modal traffic management systems. However, the high expectations of traffic management systems that are predictive rather than reactive have not been met, implying that the benefits of predicting are only demonstrated in theoretical contexts.
This is due to the complexity of the prediction task, the uncertain nature of the network-wide dynamics, and the uncertainties of the impact of traffic and mobility management interventions. At the same time, determining near-optimal network-wide solutions is a very complicated task in a real-time context due to the high computational complexity. Therefore, distributed approaches are often used to tackle the control tasks. However, optimally distributing the processing and analytics effort entailed is underdeveloped and requires a thorough understanding of complex multi-modal network dynamics. The role of data analytics is pivotal to this.
How EMERALDS will address the challenge
Cities often have many data sources at their disposal. However, processing, analysis, and use (e.g., for context-dependent prediction) is hindered by the lack of applicable methods and extreme data processing tools. EMERALDS will address this challenge by:
- Developing schemes for network-wide multi-modal estimations and predictions of multi-modal traffic operations, where multi-modal refers to an integral approach to assess the combined traffic networks of road traffic, public transport and active modes. Interactions and dependencies between modes are incorporated.
- Developing EMERALDS services to support predictive multi-modal network-wide traffic management strategies using novel data mining, aggregation, and analytics techniques.
- Investigating the potential of explainable (or interpretable) AI in complex, real-time control applications.
Making effective use of the extreme amount of data (radar data, data from vehicles, bicycle data, public transport data, loop detector data, traffic management operations status messages, weather data, and emission information) to provide answers to these problems.
How it will work
The use case focuses considers multi-modal traffic operations in the metropolitan area of Rotterdam and focuses on enabling extreme data-driven methods for predictions and the required network analysis to determine decentralization opportunities. Previous approaches have used distributed reactive methods using dedicated data collection services. In EMERALDS, we anticipate the following innovations:
- reducing the need for dedicated (and expensive) data collection methods by using advanced techniques that use existing data sources to achieve comparable information quality
- self-identification of underperformance and self-tuning, including multi-modal network decomposition
- providing multi-modal multi-sensor network estimation (e.g., AI-driven data fusion) and assessment of bottlenecks, and providing context-dependent multi-modal network predictions (short-term) for anticipatory traffic management purpose
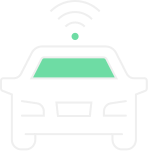