The collection and analysis of mobility data have become integral to understanding and optimising urban transportation systems. With the increasing presence of IoT sensors, GPS-enabled devices, and intelligent transport solutions, vast amounts of mobility data are being generated daily. This data holds immense potential for improving public transport efficiency, traffic management, and urban planning. However, handling such large-scale datasets presents significant challenges, particularly regarding privacy, data completeness, and processing efficiency.
Curious to learn more about data processing and analytics services in mobility data? Join our webinar on the 14th of February, from 14:00 to 15:30 CET.
Privacy Challenges in Mobility Data
Even when anonymised, mobility data can still pose privacy risks. Unique movement patterns can reveal individual identities, especially if a sequence (trajectory) of multiple data points is cross-referenced. Protecting privacy while at the same time enabling meaningful analysis is a key challenge for mobility data services.
The EMERALDS project addresses this issue by developing privacy-preserving methods to detect and eliminate compromising trajectories. One focus is assessing the applicability of these methods in edge computing environments, allowing mobility data to be processed closer to its source. By optimising privacy attack detection and data ingestion speed, EMERALDS enables scalable and secure mobility analytics.
Scalable Processing of Mobility Data
Analysing large-scale spatial data requires efficient processing techniques. One approach is parallel processing of spatial joins, a very common operation is spatial data management systems, where the involved datasets are divided into partitions to enable simultaneous computation. Traditional methods replicate objects across partitions to avoid duplicate results, but this increases computational overhead.
EMERALDS introduces an adaptive replication strategy that dynamically determines which data points need to be duplicated. This minimises redundancy, reducing computational load while improving processing speed. Implemented in the popular Apache Spark platform, this approach outperforms conventional methods, making big spatial data analysis more scalable and efficient.
Improving Data Quality and Usability
Mobility datasets often contain missing values due to sensor failures, GPS inaccuracies, or data transmission issues. Incomplete data can compromise the reliability of transport models and decision-making processes.
EMERALDS applies estimation techniques to reconstruct missing values, ensuring data integrity. Additionally, the sheer volume of mobility data necessitates efficient storage and retrieval solutions. Advanced compression techniques enable large-scale datasets to be managed effectively, reducing storage costs and improving accessibility for downstream analysis.
Predictive Analytics for Urban Mobility
Demand forecasting is a critical component of intelligent transportation systems. Understanding future movement patterns helps optimise vehicle dispatching, route planning, and congestion control. A federated learning approach allows sub-regions to retain local data while sharing only relevant insights with neighbouring areas. This method enhances prediction accuracy while maintaining data privacy.
For crowd density forecasting, a model predicting movement trends over an 11-day horizon has been implemented. By using explainability techniques like SHAP and LIME, analysts can identify key factors influencing predictions, enabling more transparent and effective urban mobility strategies.
In addition to long-term forecasting, real-time traffic density estimation plays a crucial role in adaptive transport management. EMERALDS has developed machine learning models based on gradient boosting to classify and predict demand patterns at various time scales, from five minutes to an hour. These models have been trained on real-world micro-mobility data from Dutch cities, demonstrating their effectiveness in capturing urban mobility dynamics.
Efficient data processing and analytics services are essential for transforming mobility data into actionable insights. By addressing privacy risks, improving processing scalability, and applying predictive modelling techniques, mobility data analytics can support smarter, more responsive urban transportation systems. The work performed in EMERALDS highlights the potential for innovative, privacy-preserving solutions that balance data utility with security, ultimately enhancing mobility management in modern cities.
To learn more, join our webinar on the 14th of February!
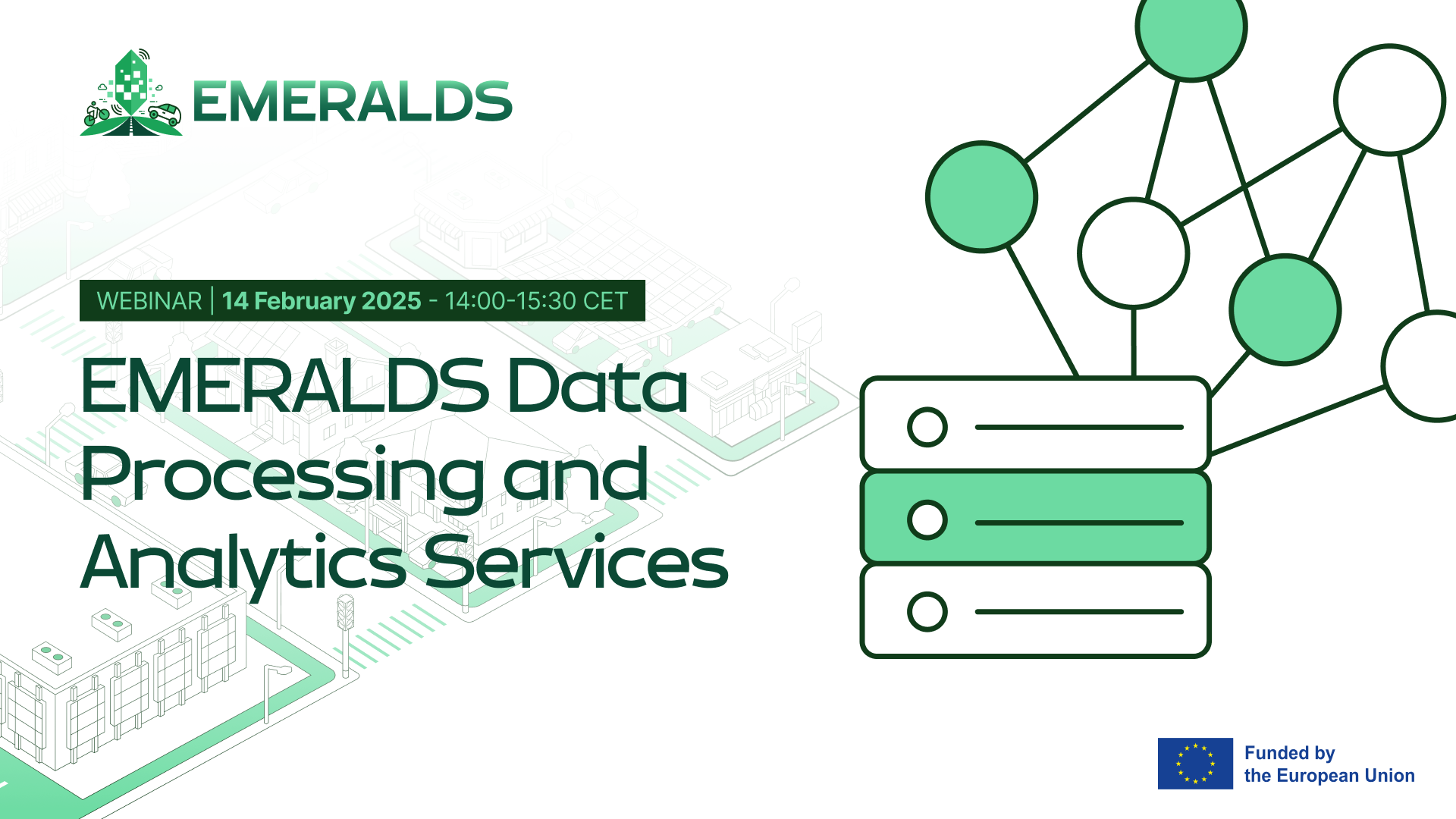