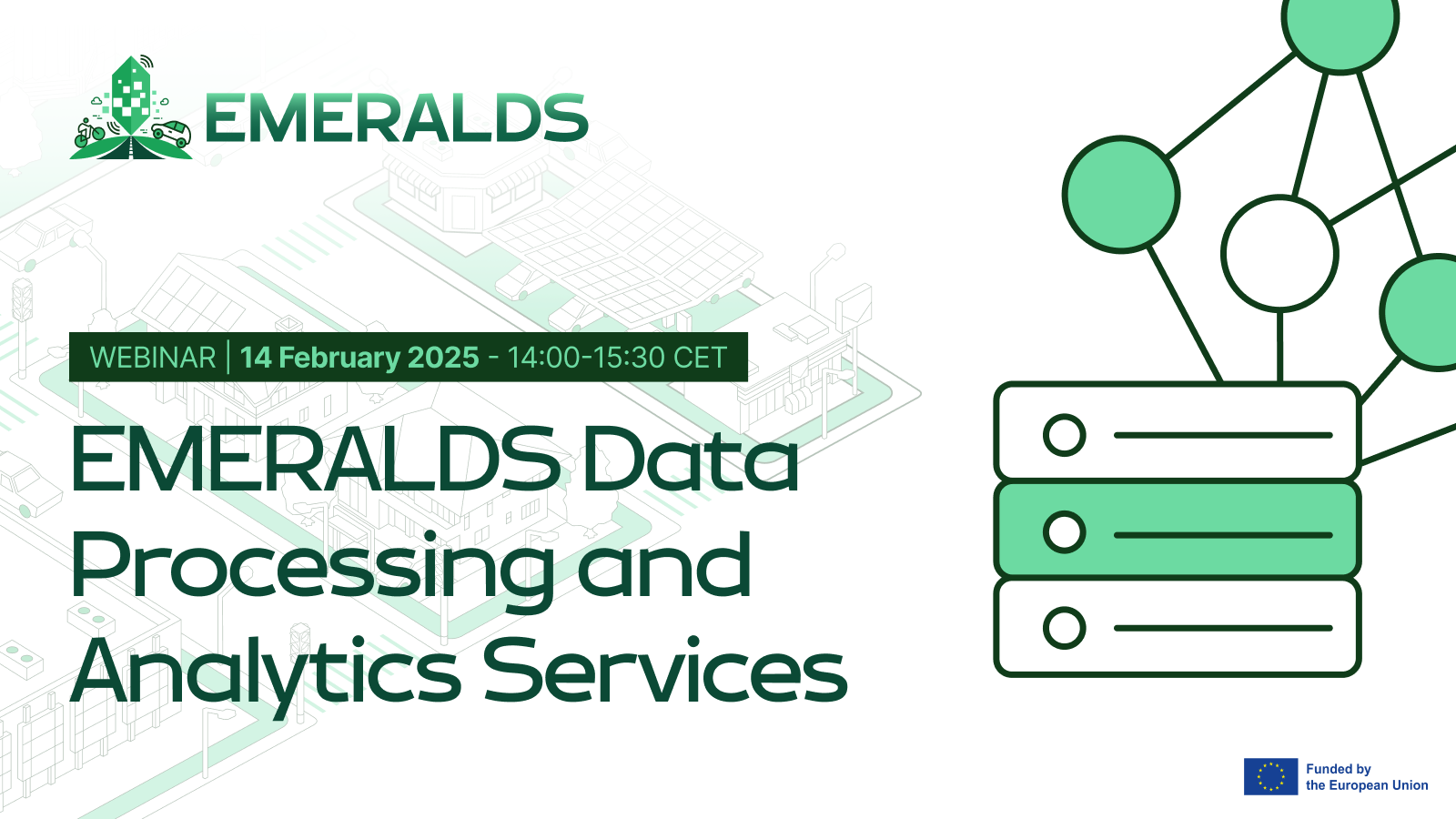
Join us on Friday, 14th of February, for our next webinar showcasing the latest innovations in mobility data management and analytics from the EMERALDS project. This session will explore new solutions to key challenges in mobility data, including addressing missing values in sparse datasets, enabling privacy-aware data ingestion, optimizing urban micro-mobility forecasting, and advancing scalable analytics for big spatial data.
Our experts will also present a federated learning framework for crowd density forecasting, combining predictive accuracy with explainability to support informed decision-making.
Don’t miss this opportunity to learn how EMERALDS is shaping the future of smart mobility systems through new research and practical applications.
Read and download the post-event report
Agenda
- 14:00 - 14:10 | Project Overview and Status - Konstantinos Dafloukas & Yannis Theodoridis
- General overview of the EMERALDS project
- 14:10 - 14:20 | Privacy-Aware Mobility Data Ingestion - George Theodoropoulos
-
In the era of ubiquitous sensors and mobile devices, human movements are being tracked at unprecedented rates.
This development significantly increases the risk to individual privacy, as unique movement patterns can potentially be used to identify individuals even when their trajectories have been anonymised.
During the EMERALDS project, UPRC has been working on a set of privacy attack methods designed to detect and eliminate such compromising trajectories. A key aspect of this work involves assessing the overall performance and applicability of these methods within the Computing Continuum, with particular emphasis on edge computing environments.
Results show a significant speedup over current implementations, paving the way for scalable and in-situ privacy-preserving mobility data ingestion. - 14:20 - 14:30 | Extreme-scale Parallel Distance Joins - Christos Doulkeridis
- Processing spatial distance joins in parallel facilitates scalable analytics of big spatial data.
The state-of-the-art algorithms rely on dividing the data into partitions and replicating objects from one data set in neighboring partitions, so that partitions can be processed in parallel independently without producing duplicate results. Instead, in the EMERALDS project, we advocate an approach that adaptively selects which data set to replicate in different local areas of the space, thus minimizing replication and boosting the performance of query processing.
We implement our algorithm based on adaptive replication in Apache Spark and demonstrate its efficiency and scalability in comparison with the state-of-the-art. - 14:30 - 14:40 | Mobility Data Fusion and Managements - Salehi Bahare
-
Mobility data is a valuable resource for understanding and optimising traffic systems, but challenges like missing values and massive data volumes often arise.
In the EMERALDS project, our team tackles these issues by focusing on the tasks of estimating missing values in sparse datasets to ensure data completeness, and applying advanced compression techniques to efficiently manage large-scale mobility data.
These efforts improve data usability and system scalability, and provide a seamless integration for downstream analysis and decision-making tasks. - 14:40 - 14:50 | Urban Micro-mobility demand forecasting - Antonis Tziorvas
-
Urban demand forecasting is essential for optimising routing, dispatching, and congestion management within Intelligent Transportation Systems.
By utilizing data fusion and analytics techniques, traffic density estimation emerges as a crucial step in identifying and predicting emerging demand patterns. UPRC has developed 2 gradient boosting models: one for classification and another for regression that are designed to generate demand forecasts across various time frames, ranging from 5 minutes to an hour, addressing the dynamic nature of urban mobility.
A real-world dataset from micro-mobility service providers that spans two Dutch cities has been used to provide a robust basis for testing of the models, demonstrating their effectiveness in capturing the complexities of modern urban mobility. - 14:50 - 15:00 | Explainable and federated crowd density forecast - Anahid Wachsenegger
-
We present a federated learning implementation for an hourly crowd density forecast of the next 11days, where data was retained locally by sub-regions, and only embeddings were shared with neighboring regions, allowing for collaborative forecasts that were significantly more accurate than isolated predictions.
Moreover, explainability techniques such as SHAP and LIME were applied to provide clear insights into the factors influencing the full-region model’s predictions.
This approach ensures improved predictive accuracy while supporting informed and effective crowd management. - 15:00 - 15:20 | Q&A Session
-
Q&A Session with the speakers to address any questions or comments the audience might have.